Profit matters most when evaluating forecasting models

The most important part of forecasting is how it improves downstream decision making relative to the status quo. For instance, how does load data inform the generation stack, or how do price forecasts optimize battery charging? As we move through the ‘forecasting stack’ from foundational weather to market-level prices, it becomes easier to measure how accuracy impacts your profit-and-loss.
When evaluating new forecasts—whether from a vendor or a model—traders often rely on a simple approach: reviewing performance during volatile periods, calculating basic metrics like MAE and RMSE, and comparing results to in-house forecasts if available. This method is quick and relies on analysts’ ability to identify critical high-leverage hours. However, more targeted evaluation methods can better align forecasts with specific use cases.
The Gold Standard: Profit and Loss
The best way to evaluate a new model or vendor is to measure how their data would have impacted decisions and the resulting change in profit. This requires significant investment in software or data preparation, along with a historical baseline for comparison. Without a record of how past forecasts influenced decisions, it’s hard to quantify the profit changes from a new approach. Advanced traders with robust systems can integrate new models into backtests to measure profit impact directly, though creating reliable backtests comes with its own challenges.
“It doesn’t matter how a model performs on a myriad of acronyms; what matters is the profit those forecasts would generate over and above your current state.”
Cumulative Profit Over Time
When models can be directly attributed to profit, the evaluation is obvious
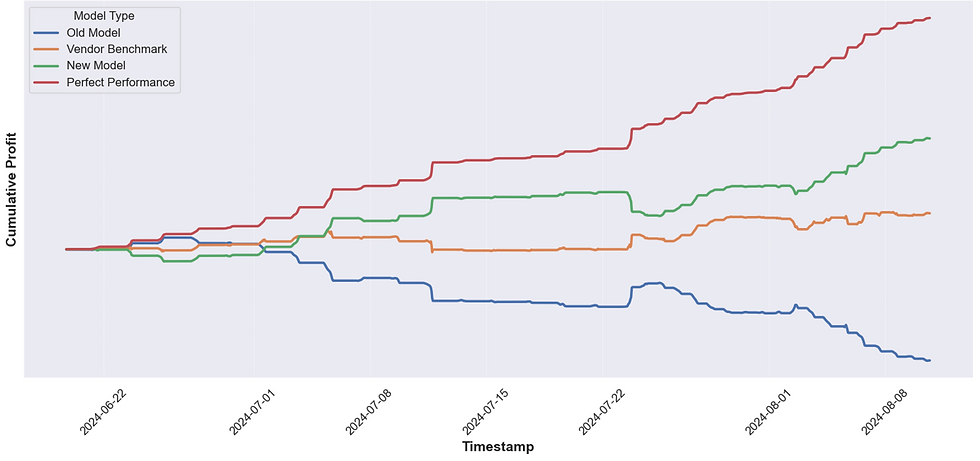
Price Volatility with Price Spike Matrices
Many users of energy forecasts count accuracy as only one of many important considerations they worry about, and do not have resources to log a system of record, modularize an evaluation pipeline for new forecasts, or directly link profits to a new model’s forecast. Typically, these users have a good sense for how prices will affect trading performance during low volatility and may be more concerned about price spikes.
In these cases, simple matrices denoting whether a price spike was forecasted plotted against whether a price spike occurred can yield very helpful insights into how a model may support an analysts decisions.
Comparing How Models Predict Price Spikes
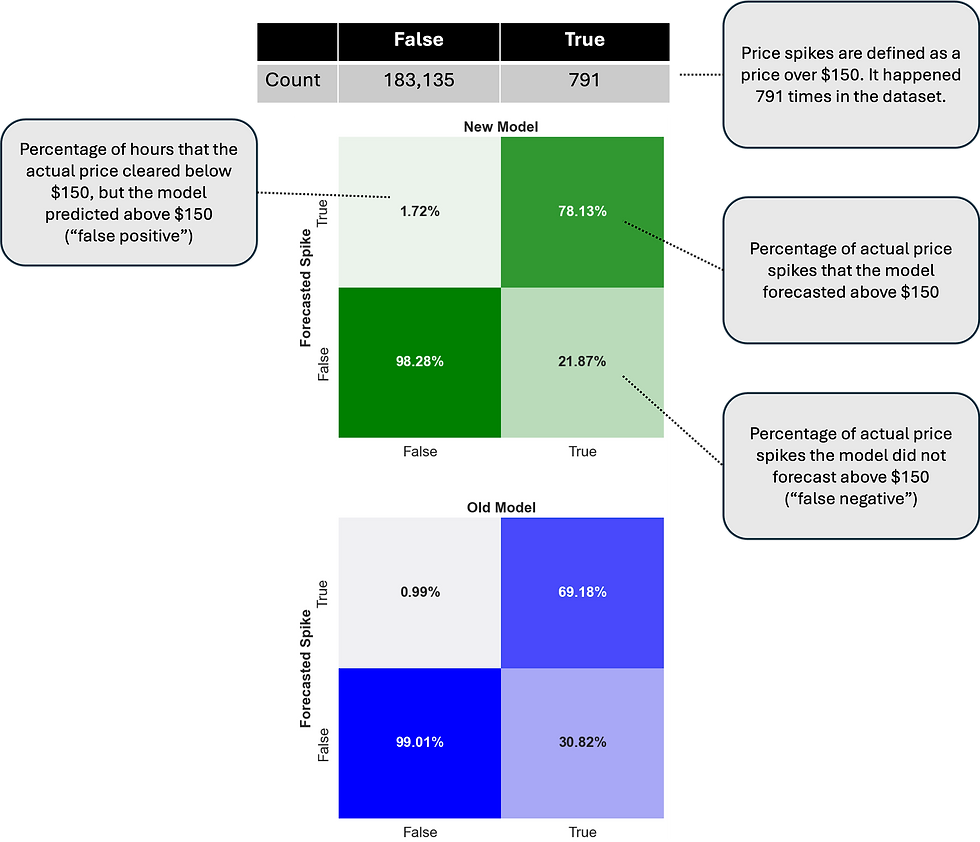
Key metrics for these evaluations are false positives and false negatives, reflecting the cost of errors. What’s the cost of predicting a large price spike that doesn’t happen (false positive)? Or forecasting mild prices when a spike occurs (false negative)? Analysts should weigh these costs and select a model that strikes the right balance.
Model Calibration and Validity for Optimization
Calibration measures how well prediction intervals match actual outcomes. In optimizations, it’s often less critical for forecasts to provide perfect point accuracy than for their distributions to reliably reflect possibilities. For instance, does the range between the 5th and 95th percentiles capture 90% of actual prices? Better-calibrated models improve optimizations based on probabilistic forecasts. Day-ahead prices are typically more stable than real-time, and models should reflect this difference in price variability.
Forecast validity focuses on band width. While predicting that 90% of prices fall between $-50 and $1000 may be technically correct, it’s not useful. Instead, forecasts should provide meaningful ranges that vary by time of day, enabling optimizations to leverage forecasted volatility effectively.
Width of Prediction Intervals and Coverage Accuracy
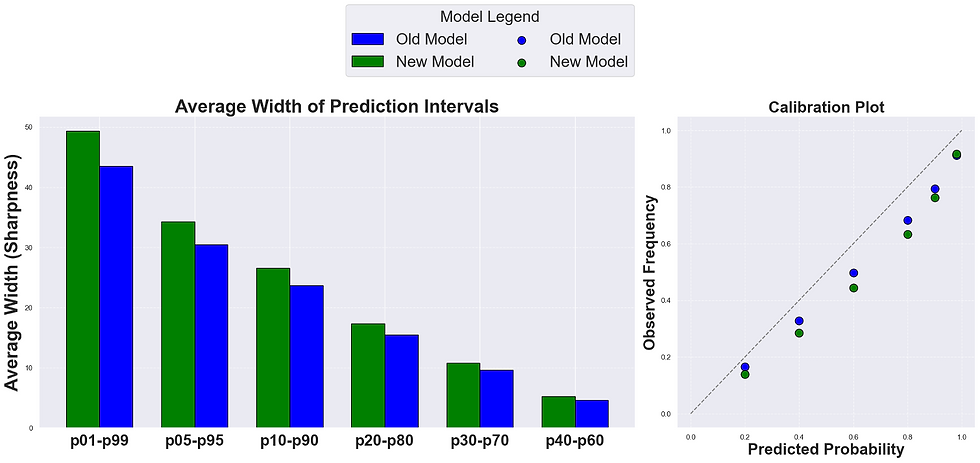
Summary
Evaluation of forecasts from new models should be catered to the use case, and not blanketed by acronyms. The gold standard is measuring the actual impact on profit and loss to assess a model’s value. For those without significant software resources, simpler methods can still provide insight. Evaluating how well a model handles price spikes is valuable if the costs of errors in either direction are clear and easy to calculate. Calibration measures how well prediction intervals align with actual outcomes, while validity reflects the usefulness of forecasted volatility.
Enertel AI provides short-term energy and ancillary price forecasts for utilities, independent power producers (IPPs), and asset developers to inform trading strategies. You can view our data catalog, view a clickthrough demo of our product for operators, or request a sample of backcast data for your assets.
Comments